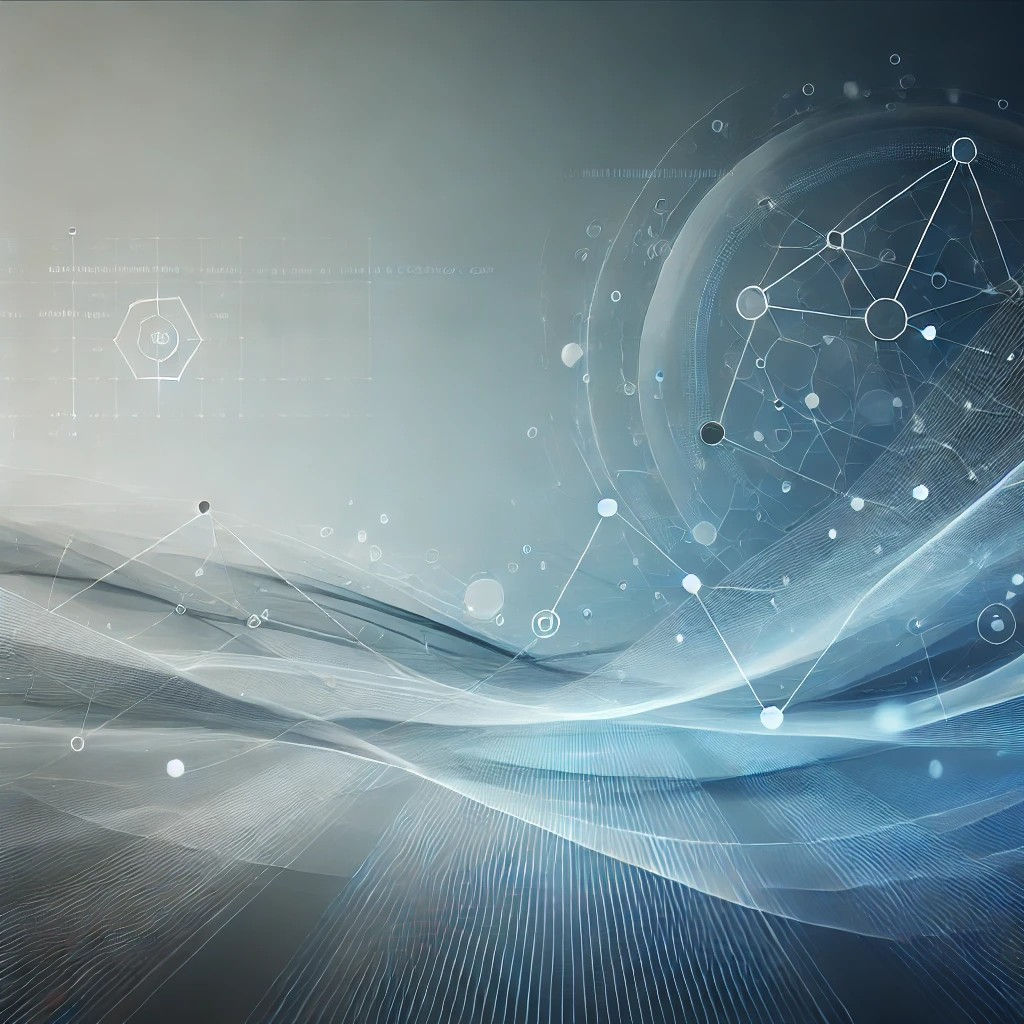
S/4HANA Implementation - ongoing
Global Digital Transformation Program, including SAP ECC to SAP S/4 HANA implementation and data migration
Challenge
Global SAP S/4HANA Implementation and data migration program involving over 20 countries and multiple business units, across all functions. The goal is to ensure seamless data integration while aligning with the organization’s digital transformation initiatives and functional requirements.
Approach
-
Develop and implement a comprehensive data migration strategy covering extraction, transformation, loading (ETL), and validation processes
-
Collaborate with cross-functional teams, including IT, business stakeholders, and external vendors (functional / technical / developers), to develop functional and technical specifications, address data gaps and align with enterprise architecture
-
Provide leadership to a geographically diverse team, offering guidance and coaching to meet tight deadlines and budget constraints
-
Conduct risk management activities, identifying potential issues early and devising mitigation strategies
Achievements
-
Enhancing data scope, quality and integrity through validation rules and processes, minimizing errors and ensuring consistency across business units (harmonization potential)
-
Strengthening alignment between migration efforts and broader digital transformation goals
-
Developed the program planning from migration perspective
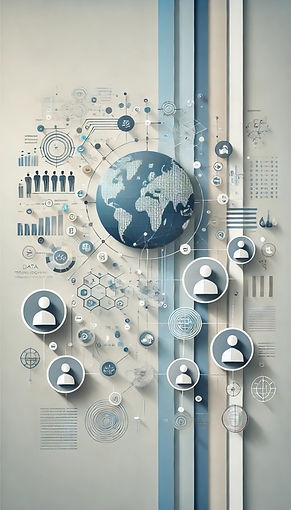
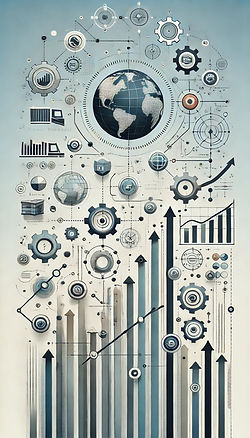
PLM Implementation
Implementation of a a new Product Lifecycle Management (PLM) solution to establish a consistent global structure for product data to maintain 100% alignment between Development and Manufacturing
Challenge
Problems in products - use of incorrect materials; use of incorrect packaging information; use of incorrect references, non-standardized processes and systems, multiple & manual interventions, offline approvals
Use of different ERPs, with different instances leading to multiple “sources of truth” that do not ensure product safety and key technical data
Approach
-
PLM rollout to R&D and SQRC connected site user base to create a system and processes to structure core product design data (e.g., Materials, BOM’s, & Specs), interface key data to ERP, “lock it in” as one version of truth, and improved data traceability
-
Establish a consistent global structure for product data and parameters and create global business processes for managing foundational ‘Product data’
Achievements
-
Up to 30% E2E cycle time improvement (increase Speed to Market)
-
Up to 5 times productivity increase by reducing reworking needs
-
Increased Data Quality and Compliance by approx. 40%
SAP ECC and MDG Implementation
Integration of the supply chain master data of a Brazilian factory into the existing SAP environment of a top 10 retail manufacturer, following a merger and acquisition. Additionally, the implementation of SAP Master Data Governance (MDG) was undertaken to ensure consistent data management and quality control
Challenge
Data Complexity: the legacy system contained incomplete or missing data structures, requiring mapping and transformation to align with the SAP data model
Data Quality: discrepancies, duplication, and inconsistencies in the legacy data
Data Governance: lack of robust governance policies and standardized data entry
Approach
-
Review of the business definitions, critical data element, technical metadata
-
Reassess the user requirements and the ability to integrate the changes
-
End-to-end testing of system integrations, automation, performance, regression, and UAT
-
SAP MDG implemented to enforce data standards, validate data entries, and establish data ownership across the supply chain master data domain
-
Review data governance policies and standards (e.g., quality, metadata, provisioning, reference)
Achievements
-
Successful Data Migration: the critical data elements were accurately transferred to the SAP system, minimizing disruption to operations
-
Enhanced Data Quality: reduced duplication, and consistent data across the supply chain
-
Operational Efficiency: the integrated supply chain master data that facilitated streamlined production planning, procurement, inventory management, and distribution

Snowflake Analytics for Advance Planning Solution
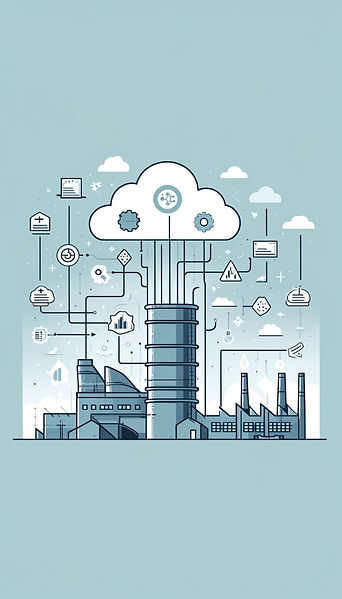
Leverage the power of Snowflake's cloud-based data warehousing and analytics capabilities to support advanced supply chain and production planning, and to publish the data for external consumption. This would have a positive impact on ability to execute decisions on allocation planning while having reliable revenue and financial forecast
Cleanse and prepare the operations and supply chain master data from both legacy systems and SAP for seamless integration into the advanced production planning tool
Challenge
Very fragmented IT landscape across factories with multiple ERP systems used, each having different instances, thereby significantly limiting a correct view and decision making of the production planning data
Lack of process standardization / optimization, missing key operations
Two distinct sets of data feed Snowflake. The data from all this sources needed to be unified and give a single global view of the production planning data
Approach
-
Conduct an in-depth analysis of the operations and supply chain master data to identify data discrepancies and inconsistencies across legacy and SAP systems
-
Complex SQL queries to extract, transform, and load data from both legacy and SAP systems into a unified format
-
Handle large datasets and complex data relationships, for data analysis, data quality checks, and to extract insights from complex datasets – up to 10 million records
-
Applied prompt engineering techniques to enhance the efficiency and performance of the SQL queries, allowing for faster data retrieval and transformation
Achievements
-
Establish a mandatory critical structure for Production Planning focused on Work Centres, Routings, BOM and Production Versions – fixed up to 80%
-
Respond quickly to changes in demand or supply chain disruptions, enabling them to adjust production schedules, inventory levels, and resource allocation, and to mitigate potential disruptions. More accurate long term demand signals
-
Achieve reduced cost, improved data quality, governance, and security, along with real-time data sharing and collaboration between different teams and stakeholders involved in supply chain and production planning. The cost benefit is derived from reduced scrap, transportation and inventory carrying costs